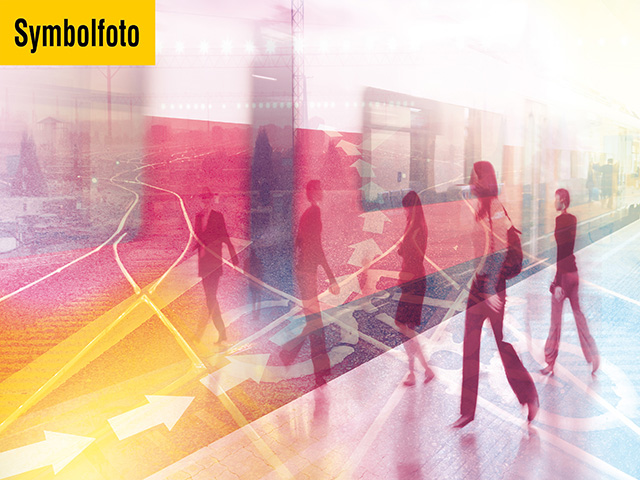
Evaluating the impact of the reduced public transport “9-euro-ticket” on air pollution, study case in Leipzig, Germany
Ziele/Ideen
Reduced-price tickets have become a common strategy to boost public transport use. However, there is a lack of knowledge and few field studies that can quantify/estimate the actual environmental benefits.
Results from a nationwide survey from the trains company Deutsche Bahn applied to 9-euro-ticket buyers indicate a reduction of 4% in the daily use of private cars/motorcycles from the pre-9-euro-ticket period to the 9-e-t period. After the campaign, everyday car use among the clients returned to the initial levels. Yet, the apparent modal shift from the survey results might be or not seen in air pollution levels. The effects of reduced tickets on private transportation and air quality differ due to multiple factors: convenience of available transport connections, habits, comfort. These aspects imply the need for specific city-based evaluation. Our goal is to use measurable variables and computational modeling to assess the ticket impacts considering the particular features of Leipzig
Kurzbeschreibung
Our research is a technology-based project aiming to evaluate the environmental impacts of the “9-euro-ticket”. The offer was available in summer 2022 and consisted of a reduced-price ticket for public transport and regional trains, valid anywhere in the country. The measure was expected to encourage people to use more public transport as an alternative to cars. Based on surveys and estimates, reports claim positive effects on air pollution and climate as a product of a modal shift. However, there is a lack of knowledge and analysis quantifying these positive environmental effects. We combine machine learning (ML) algorithms and long-term air quality and meteorology data to assess changes in pollution-related traffic emissions in Leipzig. The algorithm is trained using historical data to discount the effect of external variables such as meteorology and model expected air pollution in summer 2022. Predicted pollution is compared to the measurements and analyzed with complementary data.
Resultate
The data collection and setting up of the algorithm are completed. We established contact with local bodies such as the Dep. of General Planning and the Leipzig Transport Authority to present our research idea and collect primary/secondary data of hourly traffic counts discriminated by type of vehicle, aggregated passenger counts in public transport, and lists of construction works. Hourly air pollution data from five monitoring stations representing residential, commercial, and background environments were collected from the German Environment Agency and from our own monitoring stations. All datasets cover the period 2018-2022 and will be classified as “average” years and “pandemic” years data. We have set the algorithm and are currently working on training the model for pollutant concentrations in three monitoring stations. Next step is to apply the model to 2022 data discounting the meteorology effect and compare the results to real measured pollution and previous years data.